- Weekly AI News
- Posts
- Generative Verifiers: Reward Modeling as Next-Token Prediction
Generative Verifiers: Reward Modeling as Next-Token Prediction
Unifying Generative and Verifying Capabilities in AI: A New Approach with GenRM
Generative AI has made significant strides in recent years, offering remarkable capabilities in generating human-like text and solving complex reasoning tasks. Despite these advances, the accuracy and reliability of outputs from these models remain a challenge, particularly in critical fields like education, finance, and healthcare. Errors in these domains can have significant consequences, highlighting the need for more reliable AI systems.
Traditional Approaches: Limitations of Discriminative Reward Models
Traditional approaches to improving AI reliability involve discriminative reward models (RMs) that classify generated answers as correct or incorrect. However, these models do not fully utilize the generative strengths of large language models (LLMs). Another method, known as LLM-as-a-Judge, leverages pre-trained language models to evaluate solution correctness, but it often falls short in complex reasoning tasks requiring nuanced judgment.
Introducing GenRM: A Novel Approach to AI Verification
To address these challenges, researchers from Google DeepMind, in collaboration with the University of Toronto, MILA, and UCLA, have introduced a novel approach called Generative Reward Modeling (GenRM). GenRM redefines verification by framing it as a next-token prediction task, a fundamental capability of LLMs. This method integrates the text-generation strengths of LLMs into the verification process, allowing the model to generate and evaluate potential solutions simultaneously.

How GenRM Enhances Reasoning and Verification
The GenRM model employs a unified training approach, combining solution generation with verification. This is achieved by training the model to predict the correctness of a solution through next-token prediction, which leverages the inherent generative abilities of LLMs. The model also supports Chain-of-Thought (CoT) reasoning, where intermediate reasoning steps are generated before arriving at a final decision. This integration of reasoning and verification leads to more accurate and structured evaluations.

Performance of GenRM: Significant Improvements in Accuracy
In rigorous tests, the GenRM model, particularly when paired with CoT reasoning, significantly outperformed traditional verification methods. For instance, when verifying outputs from the Gemini 1.0 Pro model, the GenRM approach improved the problem-solving success rate from 73% to 92.8%. This substantial performance boost demonstrates the model’s ability to mitigate errors that standard verifiers often overlook.
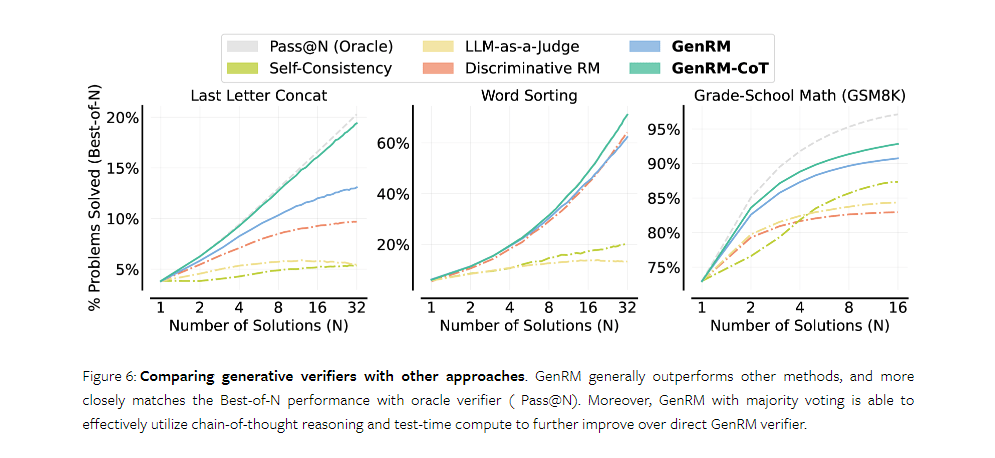
Conclusion: A New Frontier in Generative AI
In conclusion, the GenRM method represents a significant advancement in generative AI, offering a more reliable and accurate approach to solving complex problems. By unifying solution generation and verification, GenRM enhances both the accuracy of AI-generated solutions and the overall reasoning process, making it a valuable tool for future AI applications across multiple domains.
Reply